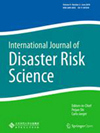
International Journal of Disaster Risk Science
INT J DISAST RISK SC
期刊介绍
The International Journal of Disaster Risk Science (IJDRS) provides a pioneering platform for researchers and practitioners aiming at greater resilience and integrated risk governance in view of local, regional, and global disasters. IJDRS breaks new ground in research about disaster risks by connecting in-depth studies of actual disasters and of specific practices of disaster risk management with investigations of the global dynamics of disaster risks and theories and models relevant for advanced integrated risk governance.
《国际灾害风险科学杂志》(International Journal of Disaster Risk Science,IJDRS)为研究人员和从业人员提供了一个开拓性的平台,旨在提高当地、区域和全球灾害的抗灾能力和综合风险治理。综合灾害风险研究系统通过将对实际灾害和灾害风险管理具体做法的深入研究与对灾害风险全球动态的调查以及与先进的综合风险治理有关的理论和模型联系起来,在灾害风险研究方面开辟了新的天地。
年发文量 | 67 |
国人发稿量 | 41 |
国人发文占比 | 61.19% |
自引率 | 6.9% |
平均录取率 | - |
平均审稿周期 | 13 Weeks |
版面费 | https://link.springer.com/journal/13753 |
偏重研究方向 | GEOSCIENCES, MULTIDISCIPLINARYMETEOROLOGY-METEOROLOGY & ATMOSPHERIC SCIENCES |
期刊官网 | https://www.springer.com/13753/?utm_medium=display&utm_source=letpub&utm_content=text_link&utm_term=null&utm_campaign=MPSR_13753_AWA1_CN_CNPL_letpb_OAXmp |
投稿链接 | https://mc03.manuscriptcentral.com/ijdrs |
质量指标占比
研究类文章占比 | OA被引用占比 | 撤稿占比 | 出版后修正文章占比 |
---|---|---|---|
94.03% | 99.48% | 0.00% | 0.00% |
相关指数
期刊预警不是论文评价,更不是否定预警期刊发表的每项成果。《国际期刊预警名单(试行)》旨在提醒科研人员审慎选择成果发表平台、提示出版机构强化期刊质量管理。
预警期刊的识别采用定性与定量相结合的方法。通过专家咨询确立分析维度及评价指标,而后基于指标客观数据产生具体名单。
具体而言,就是通过综合评判期刊载文量、作者国际化程度、拒稿率、论文处理费(APC)、期刊超越指数、自引率、撤稿信息等,找出那些具备风险特征、具有潜在质量问题的学术期刊。最后,依据各刊数据差异,将预警级别分为高、中、低三档,风险指数依次减弱。
《国际期刊预警名单(试行)》确定原则是客观、审慎、开放。期刊分区表团队期待与科研界、学术出版机构一起,夯实科学精神,打造气正风清的学术诚信环境!真诚欢迎各界就预警名单的分析维度、使用方案、值得关切的期刊等提出建议!
预警情况
查看说明
时间 | 预警情况 |
---|---|
2024年02月发布的2024版 | 不在预警名单中 |
2023年01月发布的2023版 | 不在预警名单中 |
2021年12月发布的2021版 | 不在预警名单中 |
2020年12月发布的2020版 | 不在预警名单中 |
JCR分区 WOS分区等级:Q2区
版本 | 按学科 | 分区 |
---|---|---|
WOS期刊SCI分区
WOS期刊SCI分区是指SCI官方(Web of Science)为每个学科内的期刊按照IF数值排
序,将期刊按照四等分的方法划分的Q1-Q4等级,Q1代表质量最高,即常说的1区期刊。
(2021-2022年最新版)
|
||
WATER RESOURCES | Q2 | |
GEOSCIENCES, MULTIDISCIPLINARY | Q2 | |
METEOROLOGY & ATMOSPHERIC SCIENCES | Q2 |
关于2019年中科院分区升级版(试行)
分区表升级版(试行)旨在解决期刊学科体系划分与学科发展以及融合趋势的不相容问题。由于学科交叉在当代科研活动的趋势愈发显著,学科体系构建容易引发争议。为了打破学科体系给期刊评价带来的桎梏,“升级版方案”首先构建了论文层级的主题体系,然后分别计算每篇论文在所属主题的影响力,最后汇总各期刊每篇论文分值,得到“期刊超越指数”,作为分区依据。
分区表升级版(试行)的优势:一是论文层级的主题体系既能体现学科交叉特点,又可以精准揭示期刊载文的多学科性;二是采用“期刊超越指数”替代影响因子指标,解决了影响因子数学性质缺陷对评价结果的干扰。整体而言,分区表升级版(试行)突破了期刊评价中学科体系构建、评价指标选择等瓶颈问题,能够更为全面地揭示学术期刊的影响力,为科研评价“去四唯”提供解决思路。相关研究成果经过国际同行的认可,已经发表在科学计量学领域国际重要期刊。
《2019年中国科学院文献情报中心期刊分区表升级版(试行)》首次将社会科学引文数据库(SSCI)期刊纳入到分区评估中。升级版分区表(试行)设置了包括自然科学和社会科学在内的18个大类学科。基础版和升级版(试行)将过渡共存三年时间,推测在此期间各大高校和科研院所仍可能会以基础版为考核参考标准。 提示:中科院分区官方微信公众号“fenqubiao”仅提供基础版数据查询,暂无升级版数据,请注意区分。
中科院分区
查看说明
版本 | 大类学科 | 小类学科 | Top期刊 | 综述期刊 |
---|---|---|---|---|
地球科学
2区
|
WATER RESOURCES
水资源
2区
GEOSCIENCES, MULTIDISCIPLINARY
地球科学:综合
2区
METEOROLOGY & ATMOSPHERIC SCIENCES
气象与大气科学
2区
|
否 | 否 | |
2021年12月
基础版
|
地学
2区
|
WATER RESOURCES
水资源
2区
GEOSCIENCES, MULTIDISCIPLINARY
地球科学综合
2区
METEOROLOGY & ATMOSPHERIC SCIENCES
气象与大气科学
2区
|
否 | 否 |
2021年12月
升级版
|
地球科学
2区
|
WATER RESOURCES
水资源
2区
GEOSCIENCES, MULTIDISCIPLINARY
地球科学综合
2区
METEOROLOGY & ATMOSPHERIC SCIENCES
气象与大气科学
2区
|
否 | 否 |
2020年12月
旧的升级版
|
地球科学
3区
|
WATER RESOURCES
水资源
3区
GEOSCIENCES, MULTIDISCIPLINARY
地球科学综合
3区
METEOROLOGY & ATMOSPHERIC SCIENCES
气象与大气科学
3区
|
否 | 否 |
2022年12月
最新升级版
|
地球科学
2区
|
WATER RESOURCES
水资源
2区
GEOSCIENCES, MULTIDISCIPLINARY
地球科学:综合
2区
METEOROLOGY & ATMOSPHERIC SCIENCES
气象与大气科学
2区
|
否 | 否 |